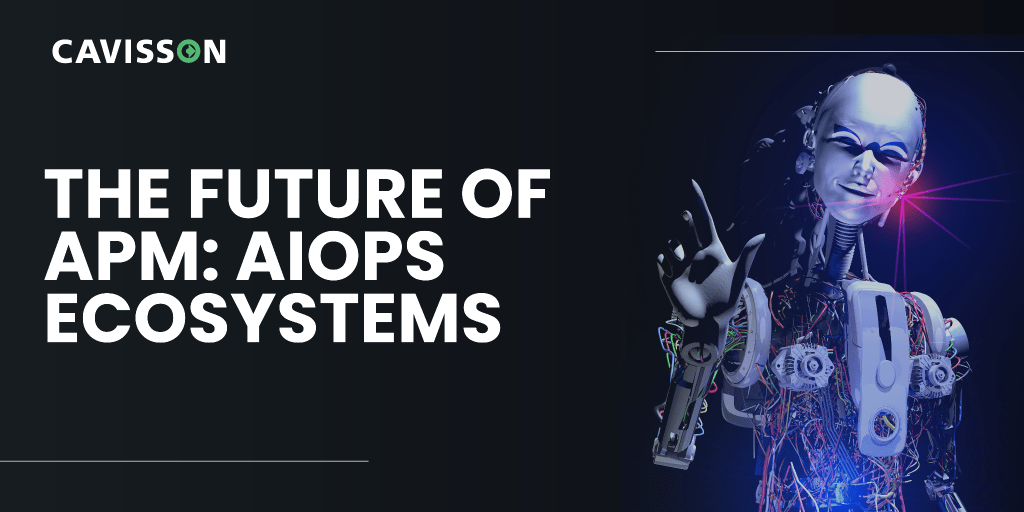
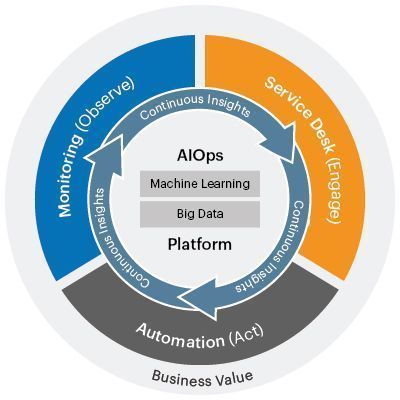
AIOps: Transforming APM Dynamics:
AIOps uses artificial intelligence and machine learning algorithms to analyze massive amounts of data, identify patterns, and predict outcomes. Organizations can move from a reactive to a proactive and predictive mindset by incorporating AIOps into APM.
This fundamental change enables several key advancements in APM capabilities:
Automated Issue Resolution: AIOps enables APM systems to automatically detect and resolve issues before they affect end users. Machine learning algorithms can use historical data to predict potential issues, allowing for proactive problem resolution. This not only reduces downtime but also increases the overall reliability of applications.
Root Cause Analysis: Identifying the root cause of performance issues has been a constant challenge. AIOps, with its ability to analyze complex relationships within the IT environment, can significantly speed up the root cause analysis procedure. This results in faster issue resolution and a shorter mean time to recovery (MTTR).
Dynamic Scalability: As modern applications scale dynamically to meet user demand, AIOps ensures that APM systems can adapt seamlessly. Machine learning algorithms can optimize resource allocation, handle traffic fluctuations, and ensure that applications scale effectively.
Enhanced User Experience: AIOps considers both the technical aspects of performance and the user experience. By analyzing user behavior and feedback, APM systems integrated with AIOps can prioritize improvements that have a direct impact on user satisfaction.
Data-Driven Decision-Making: AIOps generate a large amount of data, enabling data-driven IT operations decisions. Organizations can gain insights into trends, forecast future performance requirements, and make strategic decisions to improve their IT infrastructure.
Benefits of AIOps in APM:
Proactive Issue Resolution: AIOps ecosystems allow organizations to shift from reactive to proactive APM strategies. Using AI-driven insights, issues can be identified and resolved before they affect end users, increasing overall user satisfaction.
Operational Efficiency: AIOps uses automation and machine learning to streamline operational processes, allowing IT teams to focus on strategic initiatives rather than routine tasks. This leads to increased operational efficiency and cost savings.
Improved Collaboration: AIOps encourages collaboration among IT teams by providing a unified and comprehensive view of the entire IT landscape. This cross-functional visibility improves communication and accelerates issue resolution.
Enhanced User Experiences: The proactive nature of AIOps ecosystems ensures that applications perform consistently and optimally, resulting in better user experiences. This is especially important in industries where customer satisfaction is directly related to digital interactions.
Cavisson’s Critical AI capabilities
AutoRCA
Cavisson’s monitoring solution NetDiagnostics includes a powerful AutoRCA (automatic root cause analysis) that identifies the cause of a generated alert by analyzing all metrics captured across your entire application stack. Using the Bayesian network algorithm, millions of metrics are analyzed and a causal relationship is established to accurately identify the root cause of critical issues plaguing the application.
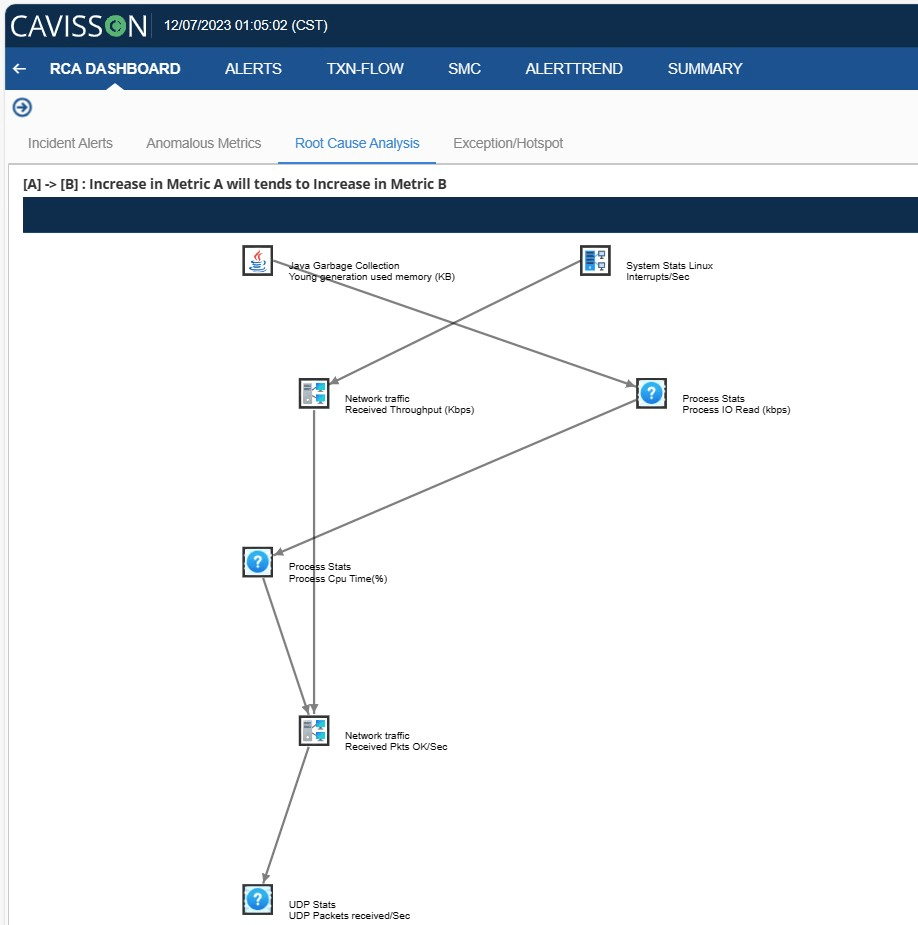
The AutoRCA feature is critical for organizations monitoring hundreds of services, thousands of hosts, and containers because it can take hours to identify the root cause. Instead of relying on manual guesswork or a time-consuming, comprehensive investigation of possible root causes, AutoRCA saves a significant amount of time, allowing organizations to focus more on delivering rather than debugging.
Outlier Detection
The AutoRCA feature is critical for organizations monitoring hundreds of services, thousands of hosts, and containers because it can take hours to identify the root cause. Instead of relying on manual guesswork or a time-consuming, comprehensive investigation of possible root causes, AutoRCA saves a significant amount of time, allowing organizations to focus more on delivering rather than debugging.
Outlier Detection
Monitoring large fleets of servers, containers, IoT devices, or application instances makes it difficult to keep track of each member’s health and performance. Cavisson’s outlier detection algorithms are constantly evaluating large fleets or groups to determine whether any member of the fleet is behaving abnormally in comparison to its peers.
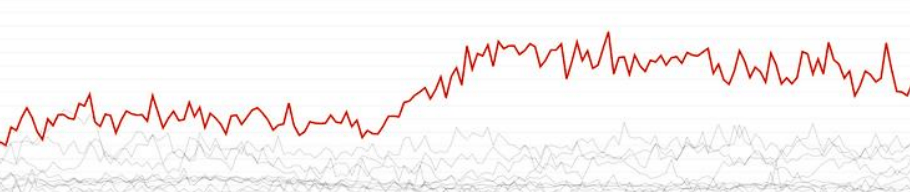
Engineers can use outlier detection to automatically identify unhealthy application servers, databases, or other systems in need of maintenance, eliminating the need to define normal, healthy behavior ahead of time.
Anomaly Detection
Anomaly detection addresses one of the most difficult challenges in monitoring dynamic, responsive, and ever-changing infrastructure: determining normal versus abnormal performance. Setting static thresholds frequently results in false alarms due to normal variations in key metrics such as website traffic and customer checkouts, which fluctuate depending on the time of day, day of the week, and month.
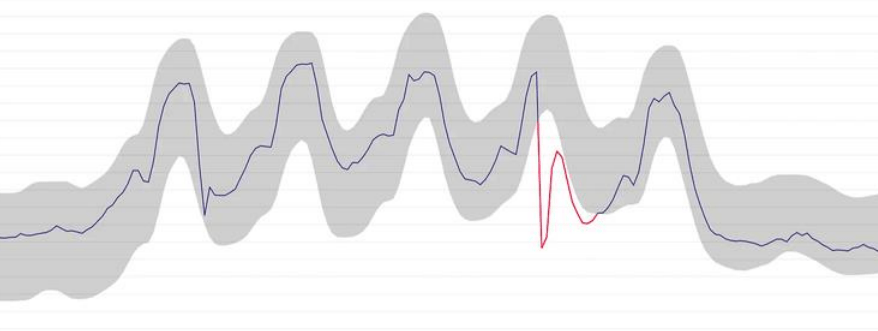
Anomaly detection takes into account both expected variations and long-term trends to intelligently identify truly unexpected behavior.
Cavisson’s anomaly detection algorithms are based on established statistical models, but they have been heavily adapted for use in high-scale infrastructure and application monitoring.
Flowpath Analyzer
An advanced debugging tool that examines the flow of transactions across multiple paths, including those with different method combinations, to identify problematic patterns and pinpoint the specific methods causing problems.
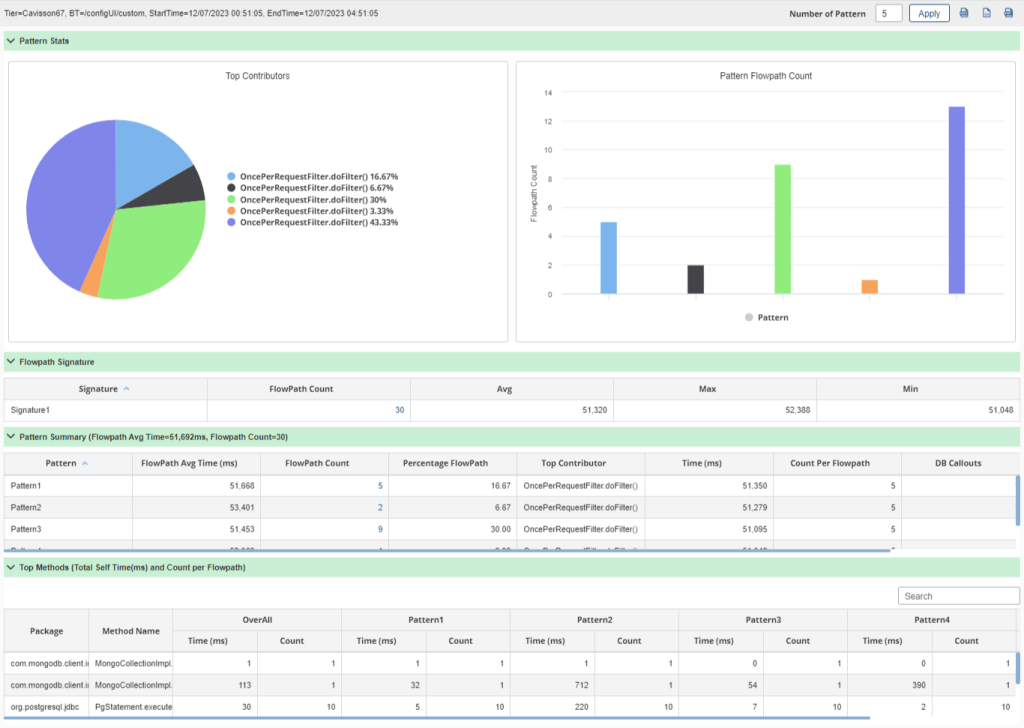
When millions of flow paths are to be assessed, the flow path analyzer uses the k-mean algorithm to identify common patterns to quickly provide extremely granular details such as top methods/packages and database call-outs, among other integral data points that are useful for IT operations in reducing MTTD and, as a result, MTTR.
Conclusion:-
The future of Application Performance Management (APM) is changing as Artificial Intelligence in Operations (AIOps) ecosystems become more integrated. AIOps, powered by machine learning and automation, shift APM from a reactive to a proactive and predictive discipline. This integration provides benefits such as automated issue resolution, faster root cause analysis, dynamic scalability, and better user experiences.
Cavisson’s advanced AI capabilities, which include AutoRCA, outlier detection, anomaly detection, and the Flowpath Analyzer, make a significant contribution to the AIOps landscape. These features improve root cause analysis, effectively monitor large fleets, intelligently detect anomalies, and provide granular insights to reduce downtime.
Ready to get started with AIOps?
Starting with AIOps is a strategic decision that promises to transform your APM. When you choose Cavisson Systems as your AIOps partner, you get not only cutting-edge AI capabilities but also a dedicated team that is committed to your success. Ready to improve your APM experience? Allow with NetDiagnostics to lead you on this transformative journey toward efficient, proactive, and predictive IT operations.